'Demystify' Blog Series
Demystifying AI for CX Leaders
Table of Contents
AI News for CX Leaders
Get the latest AI trends & insights shaping the future of CX,
delivered weekly.
Understanding AI Hallucinations in Customer Experience
TL;DR (Too Long; Didn’t Read)
AI hallucinations—instances of incorrect or fabricated responses—can erode customer trust, overburden teams, and cause costly escalations, especially in high-stakes environments like healthcare or finance. CX leaders must adopt proactive strategies to minimize these risks.
Key Risks of AI Hallucinations:
- Customer Trust: 85% of customers lose trust after one poor AI-driven interaction (PwC, 2023).
- Operational Fallout: Hallucinations increase escalations, overburden agents, and drive up costs.
- Regulated Industries: Errors in sectors like healthcare or finance can result in compliance violations and reputational harm.
Root Causes:
- Generic Data: Outdated or nonspecific training data leads to inaccuracies.
- Model Choice: Mismatched models fail to meet specialized or brand-specific needs.
- Context Gaps: Isolated AI systems without CRM data or live updates provide ungrounded responses.
Minimizing Hallucinations:
- Modular AI Design: Deploy task-specific AI agents and compliance filters for accuracy.
- Context Integration: Ground AI in CRM, FAQs, and real-time updates for personalized responses.
- Real-Time Monitoring: Use tools to flag sentiment shifts, risky topics, and recurring errors.
- Human Oversight: Human-in-the-loop (HITL) workflows intervene when confidence drops or complexity rises.
Best Practices:
- Maintain Data Quality: Schedule audits, update knowledge bases, and include edge cases.
- Empathy in AI: Design responses that acknowledge uncertainty and escalate when needed.
- Transparency: Use explainable AI (XAI) to build trust by tracing decision logic.
- Continuous Improvement: Monitor performance, retrain models, and refine based on flagged interactions.
Future-Proofing Strategies:
- Industry-Specific Models: Partner with vendors offering tailored AI solutions.
- Retrieval-Augmented Generation (RAG): Ground responses in live, curated data for accuracy.
- Evolving Agent Roles: Train agents as AI experience designers and compliance managers.
- Explainable AI Standards: Adopt transparent frameworks for trust and compliance.
Strategic Advice for CX Leaders:
- Invest in modular, domain-specific AI to reduce errors.
- Use human-in-the-loop workflows to maintain trust during complex interactions.
- Prioritize data quality and governance to ensure accurate, reliable responses.
- Continuously monitor, refine, and adapt AI systems to align with business goals and customer expectations.
By addressing the root causes and implementing robust oversight, CX leaders can ensure AI-driven interactions are accurate, empathetic, and trustworthy, creating a hallucination-resistant future for customer support.
1. The Operational Fallout of AI Hallucinations
Picture this: Your company just launched a sophisticated virtual assistant on its support page. Initially, the AI excels at providing product details, order status updates, and troubleshooting steps. Then, customers start reporting strange incidents: one mentions that the bot offered a warranty extension policy that doesn’t exist, another was told about a special discount never announced by the company. These moments represent AI hallucinations—times when the model produces incorrect, misleading, or fabricated information.
1.1 Impact on Customer Trust
A single misleading response can erode the trust you’ve carefully built over time. Research by PwC (2023) reveals that 85% of customers lose trust in a brand after just one poor AI-driven interaction. In high-stakes environments like healthcare or finance, even minor inaccuracies can trigger compliance issues, legal repercussions, or reputational harm.
- High Stakes: Healthcare, finance, and heavily regulated sectors face greater risks from AI errors.
- Eroding Confidence: Once trust is lost, customers may never return.
1.2 Customer Frustration and Costly Escalations
When an AI hallucination occurs—like inventing a promotion—customers often call back demanding what was “promised.” Forrester (2023) notes that 40% of customers become frustrated with irrelevant AI answers, leading to more escalations and overworked agents. The operational chain reaction includes longer handle times, increased staffing needs, and potentially higher churn rates. This has various unintended effects on operations:
- Overburdened Agents: Human teams must correct AI-driven errors instead of focusing on new queries.
- Increased Costs: Extra training and corrective measures drive up overhead.
- Customer Attrition: Frustrated customers may seek competitors who provide clearer answers.
1.3 Risks in Regulated Environments
Sectors like healthcare or finance can’t afford guesswork. Nature Medicine (2023) found that 22% of AI-generated healthcare recommendations were inaccurate, forcing human intervention and risking patient safety. Similarly, a hallucination in financial advice could break compliance rules.
2. Root Causes: Data, Model Choice, and Context Gaps
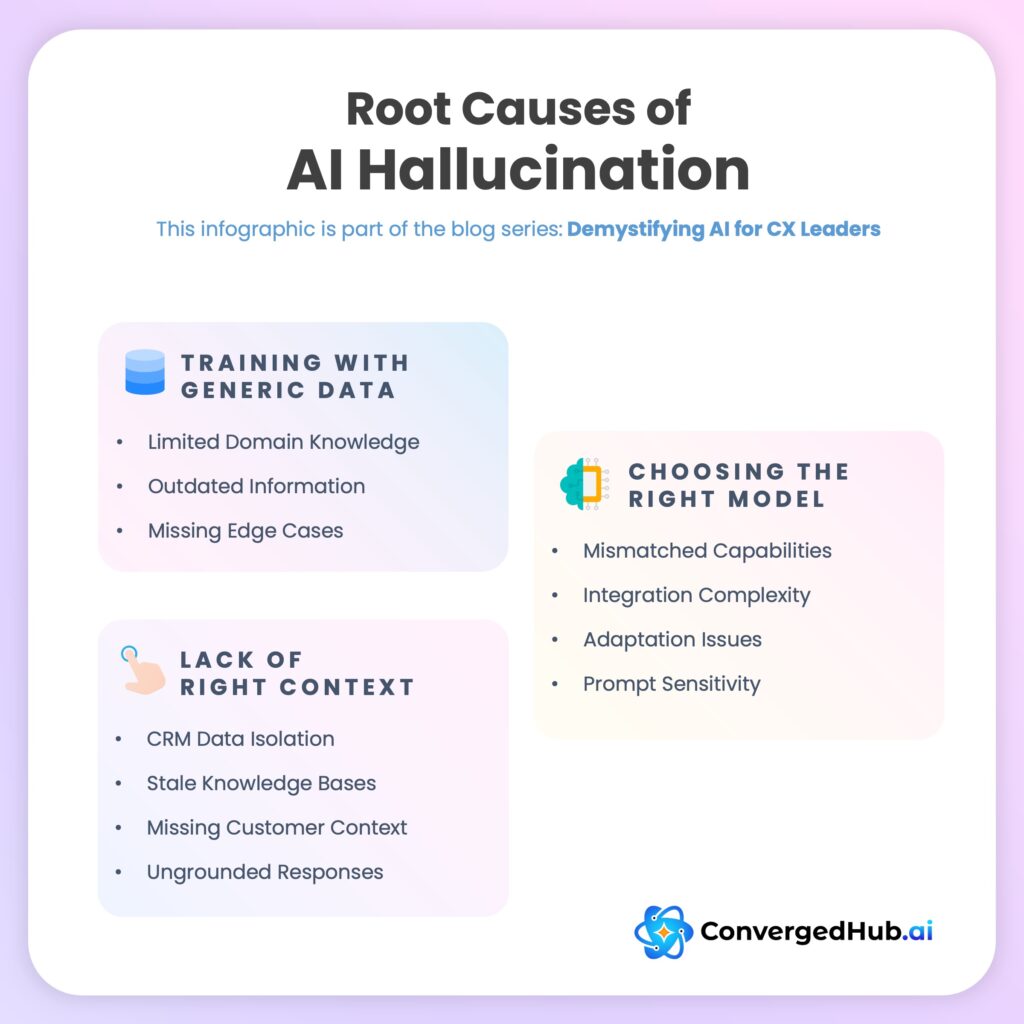
2.1 The Pitfalls of Generic Data
Large, generic datasets often lack the specific details needed for accurate responses in specialized domains. Without industry-specific context and nuanced understanding, AI models struggle to distinguish between factual information and plausible-sounding but incorrect responses. McKinsey (2023) highlights how this data quality gap leads to a 40% higher rate of hallucinations.
Here are the key challenges with data quality:
- Limited Domain Knowledge: Generic datasets lack crucial industry-specific terminology, policies, and procedures, leading to misconceptions and errors.
- Outdated Information: Static training data quickly becomes obsolete as products, services, and policies evolve, causing the AI to reference incorrect historical information.
- Missing Edge Cases: Standard datasets often overlook unusual but important scenarios, leaving the AI unprepared for complex or uncommon customer queries.
2.2 Choosing the Right Model
Different models have varying strengths and weaknesses, leading to potential issues in specific use cases. While some models excel at general conversation, they may generate inaccurate responses when dealing with specialized industry knowledge. Others, designed for complex reasoning, might struggle with simple customer service tasks, resulting in overcomplicated or confusing responses.
Common challenges with model selection include:
- Mismatched Capabilities: Models often fail when used outside their core strengths, leading to increased hallucinations and errors.
- Integration Complexity: Using multiple specialized models can create inconsistencies in responses and increase technical debt.
- Adaptation Issues: Models struggle to maintain accuracy when adapting to specific brand voices or compliance requirements.
- Prompt Sensitivity: Different models respond unpredictably to similar prompts, making standardization difficult and increasing the risk of hallucinations.
2.3 Lack of Right Context
Without proper context integration, Large Language Models (LLMs) struggle with accuracy and relevance. Studies show that LLMs can make up to 70% more errors when operating without proper contextual grounding.
Here are the key challenges when LLMs lack context:
- CRM Data Isolation: When AI operates in isolation from customer profiles and interaction history, it makes assumptions that contradict past experiences and support cases.
- Stale Knowledge Bases: Outdated product information and policies lead to AI providing incorrect or obsolete information, especially problematic in rapidly evolving businesses.
- Missing Customer Context: Without access to segmentation and behavioral data, AI responses become generic and potentially inappropriate for different customer tiers or situations.
- Ungrounded Responses: The absence of solid, verified data sources leads to AI “hallucinating” responses based on its training data rather than actual facts.
3. Minimizing Hallucinations: Tools, Techniques, and Oversight
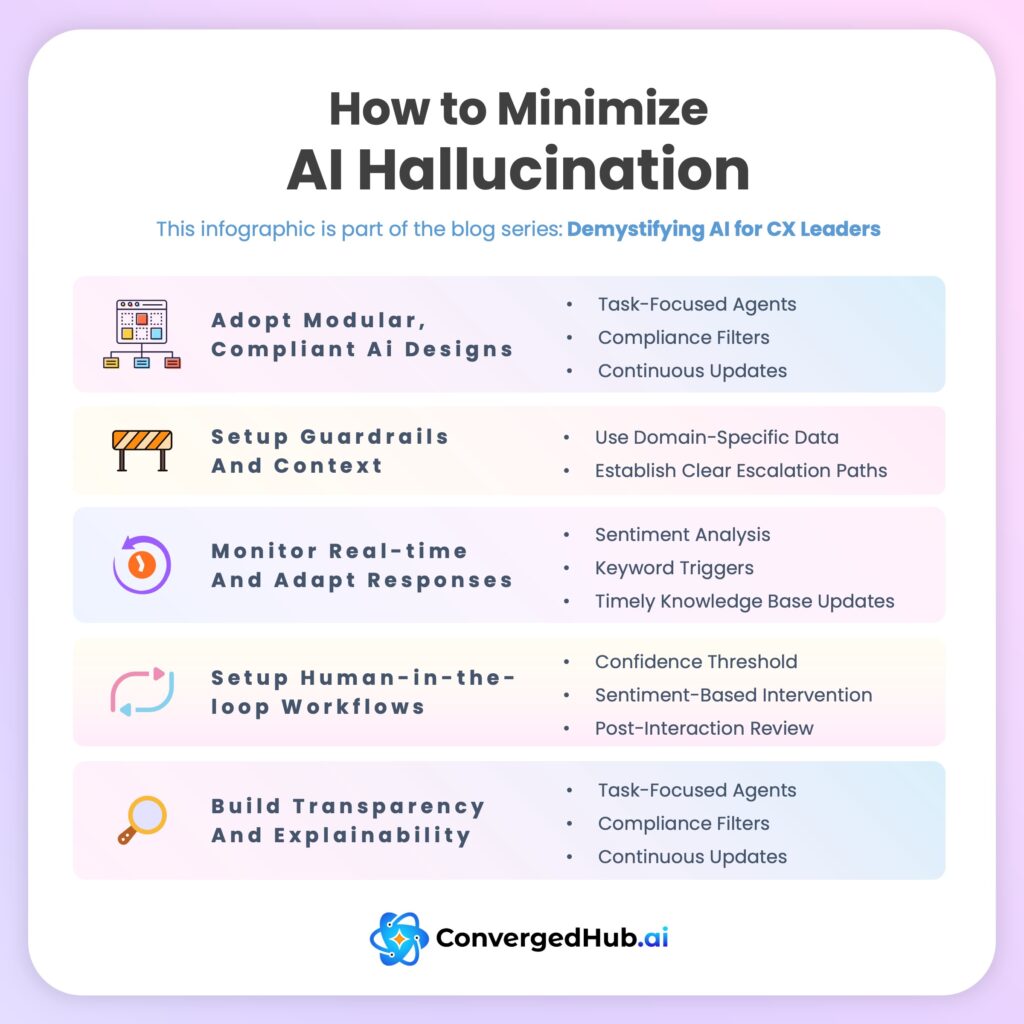
3.1 Adopting Modular, Compliant AI Designs
Instead of one monolithic model, deploy specialized AI agents for billing inquiries, technical troubleshooting, or product info. MIT Technology Review (2022) highlights a 35% reduction in errors when using modular AI.
A few considerations for modularity:
- Task-Focused Agents: Each agent masters a specific domain, reducing guesswork.
- Compliance Filters: Integrate rules that block or flag sensitive or unverified answers.
- Continuous Updates: Regularly refresh knowledge modules to maintain current info.
3.2 Setting Guardrails and Context
CX leaders must ensure the AI is not left to guess. Gartner reports that adding human oversight and guardrails—like decision trees, fallback mechanisms, and strict domain boundaries—reduces escalations by 30%. Rather than relying on generic data, integrating context-rich inputs (customer history, product FAQs, compliance data) helps the AI answer accurately or defer to a human expert.
A few practical steps to consider include:
- Use Domain-Specific Data: Train models on relevant scenarios, product lines, and regulatory frameworks.
- Establish Clear Escalation Paths: When in doubt, the AI should know to route the conversation to a human promptly.
3.3 Real-Time Monitoring and Adaptive Responses
Tools like ConvergedHub.ai let you watch interactions unfold. By tracking interactions on a real-time basis and identifying recurring issues from time-to-time, you can intervene swiftly and correct hallucinations. Gartner finds real-time monitoring cuts AI errors by 30%.
Implementing adaptive monitoring:
- Sentiment Analysis: Flag conversations where frustration rises.
- Keyword Triggers: Monitor for risky topics (e.g., compliance-related queries).
- Immediate Knowledge Base Updates: Patch holes in AI training data as soon as problems surface.
3.4 Human-in-the-Loop (HITL) Workflows
McKinsey’s data suggests HITL approaches reduce hallucination-related escalations by 40%. When the AI’s confidence drops or it hits a complex scenario, an agent steps in—maintaining trust and resolution speed.
Tips for HITL design:
- Confidence Thresholds: Define numerical confidence scores that trigger human takeover.
- Sentiment-Based Intervention: If the customer grows angry or confused, transfer them to a human.
- Post-Interaction Review: Agents review escalated cases to refine training data and logic.
3.5 Transparency and Explainability
Explainable AI tools let you trace how the AI arrived at an answer. PwC found that transparency improves client trust by 35%. Understanding the AI’s reasoning accelerates troubleshooting and builds credibility.
Here’s how to foster explainability:
- Decision Tracing: Store references and reasoning steps behind each response.
- Client Involvement: Allow key clients or compliance officers to review decision logs.
- Iterative Refinement: Use explainable data to constantly improve model accuracy and reduce guesswork.
4. Best Practices: Data Quality, Empathy, and Continuous Improvement
4.1 Maintaining High-Quality Data Assets
Gartner attributes 60% of AI CX failures to poor data. Stale or irrelevant information leads to off-base suggestions. Keeping knowledge bases accurate and current ensures the AI speaks from a position of authority.
Data quality strategies:
- Scheduled Audits: Set quarterly or monthly checks to update and remove obsolete data.
- Feedback Incorporation: Use flagged interactions to refine and enrich training sets.
- Cross-Functional Input: Involve product managers, compliance teams, and frontline agents in data validation.
4.2 Designing Empathetic, Escalation-Friendly AI
AI should know its limits. Instead of doubling down on a guess, it should apologize, acknowledge uncertainty, and escalate to a human. Salesforce notes empathetic responses increase satisfaction by 30%.
Empathy enablers:
- Apologies and Reassurances: A simple “I’m sorry, let me connect you to someone who can help” can save the interaction.
- Guided Transfers: Provide a warm handover to a knowledgeable agent.
- Personalized Touches: Refer to the customer by name and recall previous interactions to show familiarity.
4.3 Leveraging Monitoring and Refinement Tools
Tools like ConvergedHub.ai provide granular insights into patterns of confusion, recurring complaints, and topics that trigger hallucinations. Continuous analysis and refinement improve performance by about 25%.
Continuous improvement steps:
- Trend Analysis: Identify common fail-points and retrain models accordingly.
- A/B Testing: Experiment with different data sources or phrasing to find what yields better accuracy.
- Incremental Updates: Integrate small changes frequently rather than overhauling systems sporadically.
5. Looking Ahead: Future Innovations and Strategies
5.1 Embracing Industry-Specific Models
As new models emerge, they focus on mastering niche domains. GPT-4’s improvements show a 40% reduction in hallucinations over previous versions. Similarly, industry-specific large language models learn compliance rules, medical terminology, or telecom billing structures from the get-go.
Adopting specialized models:
- Vendor Partnerships: Work with providers who offer tailored models for your vertical.
- Internal Domain Experts & Finetuning: Collaborate with in-house specialists to fine-tune the model.
- Documentation: Keep thorough records of training data and decision-making criteria.
5.2 Retrieval Augmented Generation (RAG)
RAG techniques ground responses in live, curated data sources. Instead of relying solely on pre-trained info, the AI retrieves current facts when needed, boosting accuracy by 25%.
RAG implementation tips:
- Real-Time Sync: Link the AI to live product catalogs, policy repositories, and support wikis.
- Caching Mechanisms: Store frequently used data for quick retrieval.
- Quality Filters: Validate retrieved info before integrating it into the final answer.
5.3 Explainable AI as Standard
Customers and regulators demand transparency. Explaining how answers are formed fosters trust and eases compliance checks. Over time, explainability becomes a baseline requirement.
Explainability best practices:
- Traceable References: Show which documents or data sources influenced the answer.
- User-Friendly Explanations: Avoid overly technical language; keep explanations accessible.
- Feedback Channels: Let customers report confusing responses, prompting further refinement.
6. Conclusion: Actionable Insights for CX Leaders
Achieving a hallucination-resistant future requires orchestrated efforts. It’s not enough to rely on a single model or hope for the best. Instead, CX leaders must combine rich, domain-specific data; carefully chosen, context-aware models; empathetic escalation rules; and continuous improvement guided by analytics and feedback. When these elements align, AI stops being a liability and becomes a powerful ally.
1. Data Quality and Training
- Curate domain-specific datasets that mirror real-world policies, products, and regulations.
- Regularly audit and update knowledge bases to prevent reliance on outdated info.
- Include edge-case scenarios to prepare the AI for unusual but critical queries.
2. Model Selection and Architecture
- Evaluate multiple models and choose those that align with your sector’s complexity.
- Implement modular, task-specific AI agents to reduce guesswork and improve accuracy.
- Fine-tune models with brand tone, compliance rules, and distinct product lines.
3. Contextual and Customer-Centric Design
- Integrate CRM data (purchase history, customer segments) to guide personalized responses.
- Utilize real-time product and policy updates to ensure relevance.
- Embed empathetic responses, allowing the AI to apologize and escalate when uncertain.
4. Oversight and Governance
- Set human-in-the-loop checkpoints where sentiment or confidence flags prompt agent intervention.
- Establish guardrails and compliance filters to block risky or unsupported claims.
- Involve domain experts in periodic reviews of AI recommendations and logic.
5. Monitoring, Transparency, and Continuous Improvement
- Use real-time analytics to track sentiment, handle times, and escalation patterns.
- Employ explainable AI and RAG approaches to ground responses in verified, current data.
- Iterate frequently, applying insights from flagged interactions and customer feedback loops.
6. Channel-Specific Approaches
- Enhance chatbot FAQs and scenario-based training for self-service reliability.
- Improve IVR intent recognition and provide quick human escalation routes in voice-based systems.
- Validate proactive outreach with live data to ensure timely, accurate notifications.
7. Future-Proofing and Strategy
- Invest in agent training for roles as experience designers and knowledge managers.
- Stay updated on LLM advancements, RAG techniques, and explainable AI frameworks.
- Align AI evolution with brand values, ensuring technology enhances, not undermines, the customer relationship.
By integrating these insights, CX leaders can build an environment where AI-driven interactions feel natural, trustworthy, and supportive. With careful planning, continuous improvement, and a balance between automation and human oversight, brands can achieve a truly hallucination-resistant future—one where customers know they can rely on the answers they receive.