'Demystify' Blog Series
Demystifying AI for CX Leaders
Table of Content
BOOK A DEMO
ConvergedHub.AI empowers CX Leaders to build innovative AI-led Customer Experiences.
Talk to us today to learn more.
Understanding Generative AI vs. Machine Learning vs. Deep Learning
Artificial Intelligence (AI) is reshaping industries, and nowhere is this more evident than in customer experience (CX). For contact center leaders, understanding the distinctions between Generative AI, Machine Learning (ML), and Deep Learning (DL) is essential.
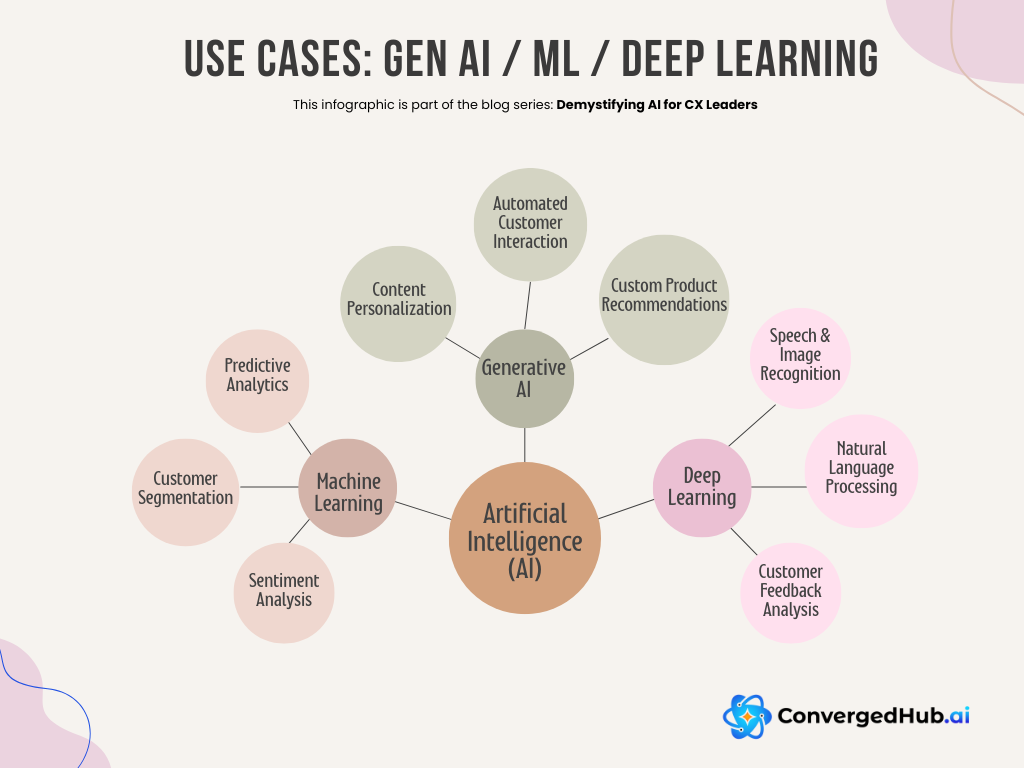
Machine Learning (ML): Foundation of Modern AI
Machine Learning is a subset of AI where systems improve performance by learning from data. ML identifies patterns, makes predictions, and adapts to new information.
Applications in Contact Centers:
Below are a few sample applications that ML can support:
- Predictive Analytics: ML analyzes customer data to forecast future behavior, enabling proactive engagement and churn reduction.
- Customer Segmentation: It identifies unique customer groups for personalized service and marketing strategies.
- Sentiment Analysis: ML deciphers customer emotions from text or voice data, helping agents respond empathetically.
Currently, 57% of companies use machine learning systems to enhance customer experience, while 49% leverage ML and AI tools to improve marketing and sales.
Industry-Specific Examples:
- Banking: Predict fraud risks by analyzing transaction patterns. ML-driven bots assist customers with account queries and payment reminders.
- Retail: Forecast inventory needs based on seasonal trends and customer preferences.
- Healthcare: Use ML to predict patient no-shows and schedule appointments more effectively.
Deep Learning (DL): Unlocking Complexity
Deep Learning, a subset of ML, uses neural networks with multiple layers to analyze large datasets. It models intricate patterns, making it ideal for tasks requiring high-dimensional data, such as image and speech recognition.
Applications in Contact Centers:
- Natural Language Processing (NLP): Enhances conversational AI, enabling accurate responses to complex customer queries.
- Speech and Image Recognition: Analyzes customer calls or verifies identities through biometrics.
- Customer Feedback Analysis: Extracts actionable insights from reviews and social media.
- Automation of Complex Tasks: Summarizes customer interactions in real-time or solves multi-step issues autonomously.
Notably, the deep learning market size is expected to increase from $17.97 billion in 2023 to $25.15 billion in 2024, reflecting a CAGR of 40.0%.
Industry-Specific Examples:
- Banking: Process loan applications faster by automating document analysis using DL.
- Insurance: Analyze damage photos to expedite claims processing.
- Travel and Hospitality: Transcribe and analyze calls to understand customer sentiments and improve service.
Generative AI: The Creative Powerhouse
Generative AI focuses on creating new content by learning patterns from existing data. It uses models like Generative Adversarial Networks (GANs) and Transformers to produce text, images, and other types of content.
Applications in Contact Centers:
- Content Personalization: Drafts tailored emails, FAQs, and marketing materials.
- Automated Customer Interaction: Generates realistic chatbot responses or pre-written replies for agents.
- Scenario Training: Simulates customer interactions for training new agents.
- Custom Product Recommendations: Offers tailored suggestions by analyzing individual preferences.
Interestingly, 92% of Fortune 500 firms have adopted generative AI technology, and the market is expected to exceed $66 billion by the end of 2024.
Industry-Specific Examples:
- Banking: Generate personalized financial advice based on spending patterns.
- Retail: Craft hyper-personalized email campaigns and product recommendations.
- Healthcare: Provide personalized follow-up instructions tailored to patient needs.
- Travel and Hospitality: Create custom travel itineraries and respond to inquiries with tailored suggestions.
Key Differences at a Glance
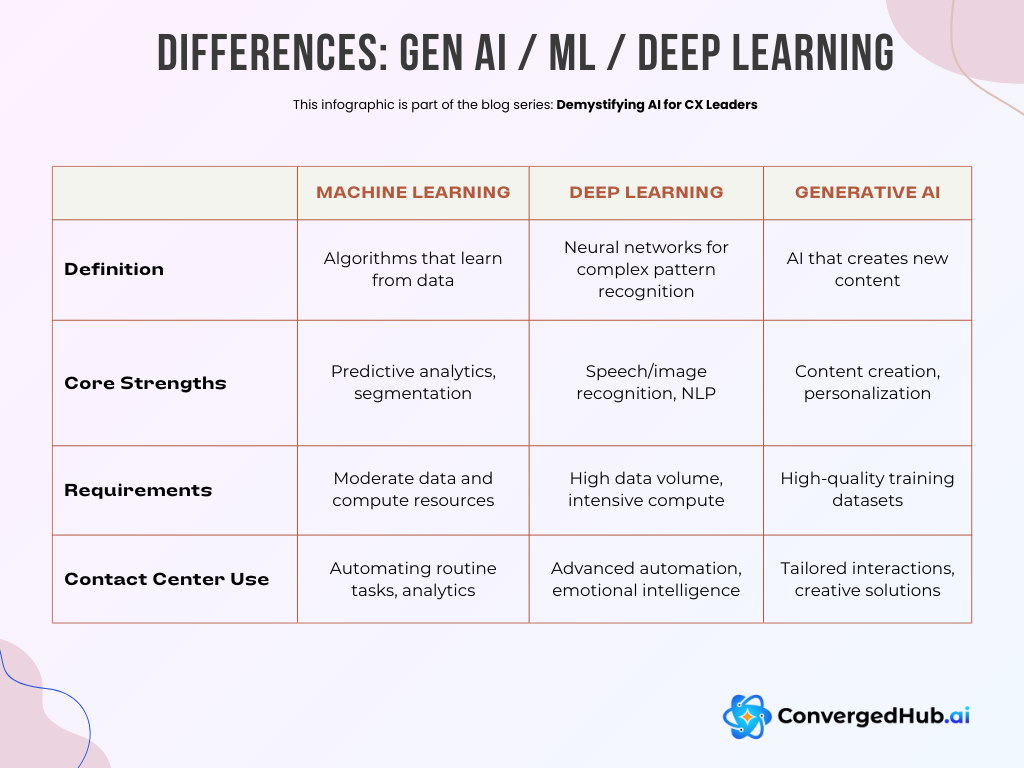
Implementation Insights for CX Leaders
- Data Quality Matters: Invest in robust data management, ensuring accuracy and regular updates.
- Ethics and Bias Mitigation: Strive for fairness and transparency, particularly with Generative AI, to build customer trust.
- Human-AI Collaboration: These technologies enhance human capabilities, allowing agents to focus on complex and value-driven tasks.
- Continuous Learning: AI models need regular refinement to remain relevant. Allocate resources for ongoing monitoring and optimization.
It’s worth noting that 85% of business leaders expect to use generative AI for low-value tasks by the end of 2024, and 45% of organizations are piloting generative AI programs.
Conclusion
For CX leaders, Machine Learning, Deep Learning, and Generative AI offer transformative opportunities across industries. Machine Learning builds the foundation with predictive capabilities, Deep Learning enables advanced understanding and automation, and Generative AI opens new possibilities for creative and personalized customer interactions. Understanding these distinctions and leveraging their strengths will position your contact center at the forefront of innovation in the AI-driven landscape.